Evans Uhunoma
DOI : https://doi.org/10.47191/ijmra/v6-i7-51Google Scholar Download Pdf
ABSTRACT:
7% of people of colour in the UK are unemployed as compared to 4% for whites (UK Gov, 2021). While recent breakthroughs in face recognition technology (FRT) have enhanced the process of recruitment through the use of on-demand- asynchronous video interview solutions (Tambe, et al., 2019; Harwell, 2019; Nawaz, 2020), several other studies have however confirmed that FRT and its application in recruitment activities are biased towards people of colour (Izario et al., 2017; Buolamwini, 2019; Simonite, 2019). Consequently, this is predicted to lead to an increased diversity crisis within the workplace as the employment of people of colour may even lessen further (West et al., 2019). The aim of this dissertation was therefore to understand how on-demand-asynchronous video interview solutions (ODAVIS) capture peculiarities in faces. Specifically, how creators of FRT determine candidates’ suitability for jobs using FRT’s facial analysis, if the data used to train their model is representative of the faces in the world and what reasonable adjustments (if any) are made for people of colour. The major findings include the use of facial landmarks and gaze estimators to analyse candidates’ facial expressions and emotions during interviews, the use of non-representative training data that leads to inconsistency in performance, and the inclusion of certain adjustments (like automatic flashlights, data augmentation or bias audit) during the designs of the solutions and implementation, to close the gaps of inconsistent performance observed for different races.
KEYWORDS:Facial Recognition Technology (FRT), On-Demand-Asynchronous Video Interview Solutions (ODAVIS), People of Colour (POC).
REFERENCES
1) Acharya, A.S., Prakash, A., Saxena, P. and Nigam, A. 2013. Sampling: Why and how of it. Indian Journal of Medical
Specialties. 4(2), pp.330-333.
2) ACLU, 2020. Wrongfully Arrested Because Face Recognition Can’t Tell Black People Apart [Online]. [Accessed 23 May
2022]. Available from: https://www.aclu.org/news/privacy-technology/wrongfully-arrested-because-face-recognition-
cant-tell-black-people-apart/
3) Ahmed, O. 2018. Artificial intelligence in HR. International Journal of Research and Analytical Reviews. 5(4), pp.971-978.
4) AI Stack Exchanges. 2019. How long it takes to train face recognition deep neural network? [Online]. [Accessed 18 July
2022]. Available from: https://ai.stackexchange.com/questions/12513/how-long-it-takes-to-train-face-recognition-
deep-neural-network-rough-estimati
5) Barragan-Jason, G., Cauchoix, M. and Barbeau, E.J. 2015. The neural speed of familiar face recognition. Neuropsychologia.
75, pp.390-401.
6) BBC, 2022. Job hunting for neurodivergent people: 'AI recruitment means I’ve got zero chance' [Online]. [Accessed 23
May 2022]. Available from:
https://www.bbc.co.uk/bbcthree/article/c62bcab6-db6f-4026-90bb-7f508705a65b
7) Bell, D. 1973. The Coming of Post-Industrial Society. New York: Basic Books.
8) Black, J.S. and Esch, V.P., 2020. AI-enabled recruiting: What is it and how should a manager use it? Business
Horizons. 63(2), pp.215-226.
9) Braun, V. and Clarke, V. 2012. Thematic analysis. APA Handbook of Research Methods in Psychology. 2, pp.57-71.
10) Bryman A. and Bell, E. 2015. Business Research Methods. 4
th ed. Oxford: Oxford University Press.
11) Brynjolfsson, E. and McAfee, A. 2014. The second machine age: Work, progress, and prosperity in a time of brilliant
technologies. New York: WW Norton & Company.
12) Buolamwini, J. 2019. Response: Racial and Gender bias in Amazon Rekognition — Commercial AI System for Analyzing
Faces. [Online]. [Accessed 09 May 2022]. Available from:
https://medium.com/@Joy.Buolamwini/response-racial-and-gender-bias-in-amazon-rekognition-commercial-ai-system-for-analyzing-faces-a289222eeced
13) Buolamwini, J. and Gebru, T. 2018. Gender shades: Intersectional accuracy disparities in commercial gender classification.
In Conference on fairness, accountability and transparency. pp. 77-91.
14) Byrne, D. 1971. The attraction paradigm. New York: Academic Press.
15) Capterra. 2022. Video Interview Software for the United Kingdom? [Online]. [Accessed 27 May 2022]. Available from:
https://www.capterra.co.uk/directory/30821/video-interviewing/software
16) Choudhury, A. 2020. 10 Face Datasets to Start Facial Recognition Projects. [Online]. [Accessed 29 May 2022]. Available
from: https://analyticsindiamag.com/10-face-datasets-to-start-facial-recognition-projects/
17) Chowdhury, M. F. 2014. Interpretivism in aiding our understanding of the contemporary social world. Open Journal of
Philosophy. 4(3). pp.327-347.
18) Choy, L.T. 2014. The strengths and weaknesses of research methodology: Comparison and complimentary between
qualitative and quantitative approaches. IOSR journal of humanities and social science. 19(4), pp.99-104.
19) Cohen, L., Manion, L. and Morrison, K. 2002. Research methods in education. London: Routledge.
20) Crawford, K. 2016. Artificial Intelligence’s White Guy Problem. The New York Times. [Online]. [Accessed 09 May 2022].
Available from:
https://www.nytimes.com/2016/06/26/opinion/sunday/artificial-intelligences-white-guy-problem.html
21) De Brito, A.C.V., Mascarenhas, A.P.F.M., de Souza, J.R., de Campos, J.A.P., Simões, M.A.C. and da Silva, R.M. 2021. Face
recognition in Service robotics: Analysis of the padding effect according to people age Reconhecimento facial em robótica
de serviço: análise do efeito de preenchimento de acordo com a idade das pessoas. Brazilian Journal of
Development, 7(12). pp.115849-115865.
22) Deloitte. 2021. Challenges of fairness in AI decisions [Online]. [Accessed 04 January 2022]. Available from:
https://ukfinancialservicesinsights.deloitte.com/post/102ftmq/challenges-of-fairness-in-ai-decisions/
23) Doug, R. 2018. Artificial Intelligence for Business: What you need to know about Machine Learning and Neural Networks,
2018. Chicago Lakeshore Press: Beaverton.
24) Duchaine, B. and Nakayama, K. 2006. The Cambridge Face Memory Test: results for neurologically intact individuals and
an investigation of its validity using inverted face stimuli and prosopagnosic participants. Neuropsychologia. 44, pp.576–
585.
25) Easterby-Smith, M., Jaspersen, L.J., Thorpe, R. and Valizade, D. 2021. Management and business research. Newcastle:
Sage.
26) Eisenhardt, K.M., Graebner, M.E. and Sonenshein, S., 2016. Grand challenges and inductive methods: Rigor without rigor
mortis. Academy of management journal. 59(4), pp.1113-1123.
27) Equality Act. 2010. Statutory Code of Practice Employment. Equality and Human Rights Commission.
28) Etikan, I. and Bala, K. 2017. Sampling and sampling methods. Biometrics and Biostatistics International Journal. 5(6),
p.00149.
29) Faux, F. and Luthon, F. 2012. Theory of evidence for face detection and tracking. International Journal of Approximate
Reasoning. 53(5), pp.728-746.
30) Forbes. 2022. Five Ways the Future Of Facial Recognition Will Become More Prevalent In Business And Life In 2022
[Online]. [Accessed 27 May 2022]. Available from:
https://www.forbes.com/sites/forbestechcouncil/2022/05/03/five-ways-the-future-of-facial-recognition-will-become-more-prevalent-in-business-and-life-in-2022
31) Francis, J.J., Johnston, M., Robertson, C., Glidewell, L., Entwhistle, V., Eccles, M.P., and Grimshaw, J.M. 2010. What is an
adequate sample size? Operationalising data saturation for theory-driven interview studies. Psychology and Health. 25,
pp.1229–1245.
32) Galterio, M.G., Shavit, S.A. and Hayajneh, T. 2018. A review of facial biometrics security for smart
devices. Computers. 7(3), p.37.
33) Gill, J. and Johnson, P. 2002. Research methods for managers. Newcastle: Sage.
34) Guo, Y., Zhang, L., Hu, Y., He, X. and Gao, J. 2016, October. Ms-celeb-1m: A dataset and benchmark for large-scale face
recognition. In European conference on computer vision. pp.87-102. Copenhagen: Springer Cham.
35) H2B. 2019. NHS Graduate Scheme Video Interview: Secure Your NHS Career! [Online]. [Accessed 28 May 2022]. Available
from: https://www.how2become.com/blog/nhs-graduate-scheme-video-interview
36) Hammersley, M. 2013. The myth of research-based policy and practice. Newcastle: Sage.
37) Han, H. and Jain, A.K. 2014. Age, Gender and Race Estimation from Unconstrained Face Images. MSU Technical Report.
[Online]. [Accessed 30 May 2022]. Available from:
http://biometrics.cse.msu.edu/Publications/Face/HanJain_UnconstrainedAgeGenderRaceEstimation_MSUTechReport2
014.pdf.
38) Harwell, D. 2019. A face-scanning algorithm increasingly decides whether you deserve the job [Online]. [Accessed 15 July
2022]. Available from: https://www.washingtonpost.com/technology/2019/10/22/ai-hiring-face-scanning-algorithm-
increasingly-decides-whether-you-deserve-job/
39) Iaydjiev, I. 2013. A pragmatic approach to social science. E-International Relations Students. [Online]. [Accessed 03 June
2022]. Available from: https://www.e-ir.info/2013/03/01/a-pragmatic-approach-to-social-science/
40) Izario, D., Izario, B., Castro, D. and Iano, Y. 2017. Face Recognition Techniques Using Artificial Intelligence for Audio-Visual
Animations. SET International Journal of Broadcast Engineering. 2017(3), pp.78-82.
41) Jia, M. 2017. Statistical Learning Methods for Facial Recognition [Online]. [Accessed 17 July 2022]. Available from:
https://openscholarship.wustl.edu/cgi/viewcontent.cgi?article=2075&context=art_sci_etds
42) Jo, S. 2018. Volvo Uses Car’s A.I. To Conduct Job Interviews [Online]. [Accessed 18 July 2022]. Available from:
https://autoconversion.net/blog/automotive/news-brief-volvo-uses-cars-a-i-to-conduct-job-interviews/
43) Kahn, J. and Bass, D. 2017. Black AI Workshop Becomes Latest Flashpoint in Tech’s Culture War. Bloomberg. [Online].
[Accessed 30 May 2022]. Available from: https://www.bloomberg.com/news/articles/2017-10-20/black-ai-workshop-
becomes-latest-flashpoint-in-tech-s-culture-war/
44) Kanade, T. 1973. Picture processing system by computer complex and recognition of human faces. Doctoral dissertation.
Kyoto: Dept. of Information Science, Kyoto Univ.
45) Kang, H.R., Yang, H.D. and Rowley, C. 2006. Factors in team effectiveness: cognitive and demographic similarities of
software development team members. Human Relations. 59, pp.1681–1710.
46) Kaur, P., Krishan, K., Sharma, S.K. and Kanchan, T. 2020. Facial-recognition algorithms: A literature review. Medicine,
Science and the Law. 60(2), pp.131-139.
47) Klein, H.K. and Myers, M.D. 1999 A set of principles for conducting and evaluating interpretive field studies in information
systems. MIS Quarterly. 23, pp.67–93.
48) Kleinman, Z. 2019. Amazon: Facial recognition bias claims are 'misleading' [Online]. [Accessed 29 May 2022]. Available
from: https://www.bbc.co.uk/news/technology-47117299
49) Kumra, S. and Manfredi, S. 2012. Managing Equality and Diversity: Theory and Practice. Oxford: Oxford University Press.
50) Lee, M. Sugiyama, U. V. Luxburg, I. Guyon, and R. Garnett. 2016. Advances in Neural Information Processing Systems.
Curran Associates. 29, pp.3315–3323.
51) Loconsole, C., Miranda, C.R., Augusto, G., Frisoli, A., and Orvalho, V. 2014. Real-time emotion recognition novel method
for geometrical facial features extraction. Proceedings of the International Conference on Computer Vision Theory and
Applications (VISAPP). pp. 378-385.
52) Lohia, P.K., Ramamurthy, K.N., Bhide, M., Saha, D., Varshney, K.R. and Puri, R. 2019. Bias mitigation post-processing for
individual and group fairness. In Icassp 2019-2019 ieee international conference on acoustics, speech and signal
processing (icassp). pp. 2847-2851). IEEE.
53) Macfarlane, B. 2010. Researching with integrity: The ethics of academic enquiry. London: Routledge.
54) Masud, M., Muhammad, G., Alhumyani, H., Alshamrani, S. S., Cheikhrouhou, O., Ibrahim, S., & Hossain, M. S. 2020. Deep
Learning-Based Intelligent Face Recognition in IoT-Cloud Environment. Computer Communications. 152, pp.215-222.
55) Meissner, P. and Wulf, T. 2017. The effect of cognitive diversity on the illusion of control bias in strategic decisions: An
experimental investigation. European Management Journal. 35(4), pp.430-439.
56) Merler, M., Ratha, N., Feris, R.S. and Smith, J.R. 2019. [Pre-Print]. Diversity in faces. IBM AI Research. New York: J. Watson
Research Centre.
57) Michael, S. 2008. The promise of appreciative inquiry as aninterview tool for field research. Development in Practice.
15(2), pp. 222-230.
58) Miller, C. 1990. Cognitive diversity within management teams: Implications for strategic decision processes and
organizational performance. Texas: Graduate School of Business, University of Texas.
59) Miller, C., Burke, L., & Glick, W. 1998. Cognitive diversity among upper echelon executives: implications for strategic
decision processes. Strategic Management Journal. 19, pp.39-58.
60) Myers, M.D. 2019. Qualitative research in business and management. Newcastle: Sage.
61) Nawaz, N. 2020. Artificial intelligence applications for face recognition in recruitment process. Journal of Management
Information and Decision Sciences. 23, pp.499-509.
62) Ness, I. 2021. Mind the Gap: Creative Knowledge Processes Within Interdisciplinary Groups in Organizations and Higher
Education. Creativity and Learning. 9, pp.195-217.
63) New York Times. 2018. Facial Recognition Is Accurate, if You’re a White Guy - Lohr, S [Online]. [Accessed 26 May 2022].
Available from: https://www.nytimes.com/2018/02/09/technology/facial-recognition-race-artificial-intelligence.html
64) Nguyen, B.T., Trinh, M.H., Phan, T.V. and Nguyen, H.D. 2017, April. An efficient real-time emotion detection using camera
and facial landmarks. In 2017 seventh international conference on information science and technology (ICIST). pp. 251-
255
65) Nguyen, L.S., Frauendorfer, D., Mast, M.S. and Gatica-Perez, D. 2014. Hire me: Computational inference of hirability in
employment interviews based on nonverbal behavior. IEEE transactions on multimedia, 16(4). pp.1018-1031.
66) Noon, M. 2010. The shackled runner: Time to rethink positive discrimination? Work, Employment and Society. 24(4),
pp.728–739.
67) Ortiz, E.G. and Becker, B.C. 2014. Face recognition for web-scale datasets. Computer Vision and Image
Understanding. 118, pp.153-170.
68) Parayitam, S., and Papenhausen, C. 2016. Agreement-seeking behavior, trust, and cognitive diversity in strategic decision-
making teams: Process conflict as a moderator. Journal of Advances in Management Research. 13(3), pp.292-315.
69) Parkhi, O.M., Vedaldi, A. and Zisserman, A., 2015. Deep face recognition. Oxford: University of Oxford.
70) Patton, M.Q. 2014. Qualitative research and evaluation methods: Integrating theory and practice. Newcastle: Sage
publications.
71) RecFaces. 2021. What Is AI Facial Recognition Tech and How does It Work? [Online]. [Accessed 27 May 2022]. Available
from: https://recfaces.com/articles/ai-facial-recognition
72) Recruiter. 2019. The rise of the Robo-recruiter [Online]. [Accessed 26 May 2022]. Available from:
https://recruiter.co.uk/2019/12/rise-robo-recruiter
73) Robson, C. 2002. Real World Research. Oxford: Blackwell.
74) Sabel, J.M. 2018. One-Way, On-Demand and Self-Paced Interview Guide [Online]. [Accessed 28 May 2022]. Available
from: https://www.hirevue.com/blog/hiring/video-interviewing-guide
75) Sardar, S., Tewari, G. and Babu, K.A. 2011, November. A hardware/software co-design model for face recognition using
cognimem neural network chip. In 2011 International Conference on Image Information Processing. pp. 1-6).
76) Saunders, M., Lewis, P. and Thornhill, A. 2007. Research methods for business students. 4th ed. London: Pearson.
77) Saunders, M., Lewis, P. and Thornhill, A. 2012. Research methods for business students. 6th ed. Harlow: Pearson
Education Limited.
78) Saunders, M., Lewis, P. and Thornhill, A. 2021. Research methods for business students. 8th ed. London: Pearson.
79) Shead, S. 2019. Google A.I. Panel Member Says Google ‘Pulled The Plug Rather Than Defend Themselves’. Forbes.
[Online]. [Accessed 30 May 2022]. Available from:
https://www.forbes.com/sites/samshead/2019/04/05/google-a-i-
panel-member-says-google-pulled-the-plug-rather-than-defendthemselves/#27d051439554
80) Shell. 2022. 7 tips to prepare students & graduates for an online video interview? [Online]. [Accessed 28 May 2022].
Available from: https://www.shell.com/careers/candidate-resource-centre/seven-tips-to-prepare-you-for-an-online-
interview.html
81) Simonite, T. 2019. The Best Algorithms Struggle to Recognize Black Faces Equally. [Online]. [Accessed 09 May 2022].
Available from:
https://www.wired.com/story/best-algorithms-struggle-recognize-black-faces-equally/
82) Statista. 2022. Poverty rate in the United States in 2020, by ethnic group [Online]. [Accessed 19 July 2022]. Available
from: https://www.statista.com/statistics/200476/us-poverty-rate-by-ethnic-group/
83) Tambe, P., Cappelli, P. and Yakubovich, V. 2019. Artificial intelligence in human resources management: Challenges and
a path forward. California Management Review. 61(4), pp.15-42.
84) Tatli, A. and Özbilgin, M.F. 2012. An emic approach to intersectional study of diversity at work: A Bourdieuan
framing. International Journal of Management Reviews. 14(2), pp.180-200.
85) Taylor, S.J., Bogdan, R. and DeVault, M. 2015. Introduction to qualitative research methods: A guidebook and resource.
John Wiley and Sons.
86) The Guardian. 2018. Psychologists' face off reveals humans can recognise 5,000 people. [Online]. [Accessed 29 May
2022]. Available from: https://www.theguardian.com/science/2018/oct/10/how-many-faces-average-person-
recognises-5000
87) Thomas, J.R., Martin, P., Etnier, J. and Silverman, S.J. 2022. Research methods in physical activity. Oxford: Human kinetics.
88) UK Gov. 2021. Unemployment: Main facts and figures [Online]. [Accessed 22 June 2022]. Available from:
https://www.ethnicity-facts-figures.service.gov.uk/work-pay-and-benefits/unemployment-and-economic-
inactivity/unemployment/latest
89) United Nations. 2020. Bias, racism and lies facing up to the unwanted consequences of AI [Online]. [Accessed 05 January
2022]. Available from: https://news.un.org/en/story/2020/12/1080192/
90) Weichselbaumer, D. 2016. Discrimination against female migrants wearing headscarves. Institute of Labor Economics.
Bonn: IZA Discussion Paper.
91) West, S.M., Whittaker, M. and Crawford, K., 2019. Discriminating systems. AI Now. [Online]. [Accessed 09 May 2022].
Available from:
https://cdn.vox-cdn.com/uploads/chorus_asset/file/16125391/discriminating_systems_041519_2.pdf
92) Wilkinson, M. 2021. The 6 Different Types of Interviews [Online]. [Accessed 04 January 2022]. Available from:
https://www.coburgbanks.co.uk/blog/assessing-applicants/6-different-types-of-interview/
93) Williams, B.A. 2017. Tech’s Troubling New Trend: Diversity Is in Your Head. The New York Times. [Online]. [Accessed 09
May 2022]. Available from: https://www.nytimes.com/2017/10/16/opinion/diversity-tech-women-silicon-valley.html
94) Wilmer, J. B., Germine, L., Chabris, C. F., Chatterjee, G., and Williams, M., Loken, E. 2010. Human face recognition ability
is specific and highly heritable. Proc. Nat. Acad. Sci (PNAS). 107(11), pp.5238–5241.
95) Younis, R. 2019. Cognitive diversity and creativity: the moderating effect of collaborative climate. International Journal
of Business and Management. 14(1), pp.159-168.
96) Zadeh, S. 2018. Are there universal expressions of emotion? [Online]. [Accessed 29 May 2022]. Available from: YouTube
https://www.youtube.com/watch?v=-hr58Yu0yDs
97) Zhang, X., Fang, Z., Wen, Y., Li, Z. and Qiao, Y., 2017. Range loss for deep face recognition with long-tailed training data.
In Proceedings of the IEEE International Conference on Computer Vision. pp. 5409-5418.
98) Zhao, J., Wang, T., Yatskar, M., Ordonez, V. and Chang, K.W. 2017. Men also like shopping: Reducing gender bias
amplification using corpus-level constraints. arXiv preprint arXiv:1707.09457.
VOLUME 06 ISSUE 07 JULY 2023
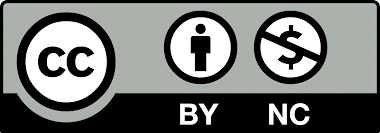
There is an Open Access article, distributed under the term of the Creative Commons Attribution – Non Commercial 4.0 International (CC BY-NC 4.0) (https://creativecommons.org/licenses/by-nc/4.0/), which permits remixing, adapting and building upon the work for non-commercial use, provided the original work is properly cited.
Our Services and Policies
Authors should prepare their manuscripts according to the instructions given in the authors' guidelines. Manuscripts which do not conform to the format and style of the Journal may be returned to the authors for revision or rejected.
The Journal reserves the right to make any further formal changes and language corrections necessary in a manuscript accepted for publication so that it conforms to the formatting requirements of the Journal.
International Journal of Multidisciplinary Research and Analysis will publish 12 monthly online issues per year,IJMRA publishes articles as soon as the final copy-edited version is approved. IJMRA publishes articles and review papers of all subjects area.
Open access is a mechanism by which research outputs are distributed online, Hybrid open access journals, contain a mixture of open access articles and closed access articles.
International Journal of Multidisciplinary Research and Analysis initiate a call for research paper for Volume 07 Issue 05 (May 2024).
PUBLICATION DATES:
1) Last Date of Submission : 26 May 2024 .
2) Article published within a week.
3) Submit Article : editor@ijmra.in or Online
Why with us
1 : IJMRA only accepts original and high quality research and technical papers.
2 : Paper will publish immediately in current issue after registration.
3 : Authors can download their full papers at any time with digital certificate.
The Editors reserve the right to reject papers without sending them out for review.
Authors should prepare their manuscripts according to the instructions given in the authors' guidelines. Manuscripts which do not conform to the format and style of the Journal may be returned to the authors for revision or rejected. The Journal reserves the right to make any further formal changes and language corrections necessary in a manuscript accepted for publication so that it conforms to the formatting requirements of the Journal.