1Mohamed Fazil, 2Rohan S, 3Ashritha C, 4Nagesh Shetty, 5Ramalingam H M
1,2,3,4,5Department of Electronics and Communication Engineering, Mangalore Institute of Technology & Engineering Mangalore, Karnataka, India
DOI : https://doi.org/10.47191/ijmra/v5-i5-06Google Scholar Download Pdf
ABSTRACT:
Agricultural production involves cultivating plants and raising domesticated animals to produce food and feed for humans and other animals. Agriculture or farming as it is also called is a complex activity, and each aspect of it impacts the overall crop production. Farmers need to manage all segments of crop production to achieve success. Farmers make strenuous efforts to produce good quality crops but they face challenging issues of monitoring and maintaining it around the clock. The problems in the agriculture domain largely affect the food production and supply chain. This project includes the proposal of an integrated crop management system to maintain the health of crops by supplying the required amount of water and nutrients to them. One aim is to reduce the amount of water lost to unnecessary evaporation, a concern in the 21st century. As a result, other factors, such as cost, time, and effective care are also benefited. This is why, after the soil preparation and planting are completed, the growth phase of the plant also requires special attention. The paper discusses a process for detecting and solving plant health issues using an intelligent automated system.
KEYWORDS:Plant Disease detection; Machine learning; Automated Irrigation; Raspberry Pi.
REFERENCES
1) R. Kanmani, S. Muthulakshmi, K. S. Subitcha, M. Sriranjani, R. Radhapoorani, and N. Suagnya, “Modern Irrigation System using Convolutional Neural Network,” 2021 7th Int. Conf. Adv. Comput. Commun. Syst. ICACCS 2021, pp. 592–597, 2021, DOI: 10.1109/ICACCS51430.2021.9441917.
2) L. Li, S. Zhang, and B. Wang, “Plant Disease Detection and Classification by Deep Learning - A Review,” IEEE Access, vol. 9, no. CCV, pp. 56683–56698, 2021, DOI: 10.1109/ACCESS.2021.3069646.
3) W. M. Ridwan, M. Sapitang, A. Aziz, K. F. Kushiar, A. N. Ahmed, and A. El-Shafie, “Rainfall forecasting model using machine learning methods: Case study Terengganu, Malaysia,” Ain Shams Eng. J., vol. 12, no. 2, pp. 1651–1663, 2021, DOI: 10.1016/j.asej.2020.09.011.
4) Y. Ai, C. Sun, J. Tie, and X. Cai, “Research on recognition model of crop diseases and insect pests based on deep learning in harsh environments,” IEEE Access, vol. 8, pp. 171686–171693, 2020, DOI: 10.1109/ACCESS.2020.3025325.
5) Ahmad, Mobeen, Muhammad Abdullah, Hyeonjoon Moon, and Dongil Han. "Plant disease detection in imbalanced datasets using efficient convolutional neural networks with stepwise transfer learning." IEEE Access 9 (2021): 140565-140580.
6) “Sunil, C. K., C. D. Jaidhar, and Nagamma Patil. "Cardamom Plant Disease Detection Approach Using EfficientNetV2." IEEE Access 10 (2021): 789-804.”.
7) S. Cramer, M. Kampouridis, A. A. Freitas, and A. K. Alexandridis, “An extensive evaluation of seven machine learning methods for rainfall prediction in weather derivatives,” Expert Syst. Appl., vol. 85, pp. 169–181, 2017,
DOI: 10.1016/j.eswa.2017.05.029.
8) C. A. T. Tee, Y. X. Teoh, P. L. Yee, B. C. Tan, and K. W. Lai, “Discovering the Ganoderma Boninense Detection Methods Using Machine Learning: A Review of Manual, Laboratory, and Remote Approaches,” IEEE Access, vol. 9, pp. 105776–105787, 2021, DOI: 10.1109/ACCESS.2021.3098307.
9) Guo, Wei, Tao Zeng, Tao Huang, and Yu-Dong Cai. "Disease cluster detection and functional characterization." IEEE Access 8 (2020): 141958-141966.
10) Huang, Shuangjie, Guoxiong Zhou, Mingfang He, Aibin Chen, Wenzhuo Zhang, and Yahui Hu. "Detection of peach disease image based on asymptotic non-local means and PCNNIPELM." IEEE Access 8 (2020): 136421-136433.
11) Zhou, C., Zhang, Z., Zhou, S., Xing, J., Wu, Q., & Song, J. (2021). Grape Leaf Spot Identification Under Limited Samples by Fine Grained-GAN. IEEE Access, 9, 100480100489.
12) Jiang, Peng, Yuehan Chen, Bin Liu, Dongjian He, and Chunquan Liang. "Real-time detection of apple leaf diseases using deep learning approach based on improved convolutional neural networks." IEEE Access 7 (2019): 59069-59080.
13) U. Ahmed, J. C. W. Lin, G. Srivastava, and Y. Djenouri, “A nutrient recommendation system for soil fertilization based on evolutionary computation,” Comput. Electron. Agric., vol. 189, no. February, p. 106407, 2021, DOI: 10.1016/j.compag.2021.106407.
14) A. Y. Barrera-Animas, L. O. Oyedele, M. Bilal, T. D. Akinosho, J. M. D. Delgado, and L. Akanbi, “Rainfall prediction: A comparative analysis of modern machine learning algorithms for time-series forecasting,” Mach. Learn. with Appl., vol. 7, p. 100204, Mar. 2022, doi: 10.1016/j.mlwa.2021.100204.
15) Zhou, Guoxiong, Wenzhuo Zhang, Aibin Chen, Mingfang He, and Xueshuo Ma. "Rapid detection of rice disease based on FCM-KM and faster R-CNN fusion." IEEE Access 7 (2019): 143190-143206.
16) N. Komal Kumar, D. Vigneswari, and C. Roth, “An Effective Moisture Control based Modern Irrigation System (MIS) with Arduino Nano,” 2019 5th Int. Conf. Adv. Comput. Commun. Syst. ICACCS 2019, pp. 70–72, 2019, DOI: 10.1109/ICACCS.2019.8728446.
17) J. Angelin Blessy and A. Kumar, “Smart irrigation system techniques using artificial intelligence and IoT,” Proc. 3rd Int. Conf. Intell. Commun. Technol. Virtual Mob. Networks, ICICV 2021, no. Icicv, pp. 1355–1359, 2021, doi: 10.1109/ICICV50876.2021.9388444.
18) K. K. Namala, A. V. Krishna Kanth Prabhu, A. Math, A. Kumari, and S. Kulkarni, “Smart irrigation with embedded system,” IEEE Bombay Sect. Symp. 2016 Front. Technol. Fuelling Prosper. Planet People, IBSS 2016, 2016, DOI: 10.1109/IBSS.2016.7940199.
19) V. Singh and A. K. Misra, “Detection of plant leaf diseases using image segmentation and soft computing techniques,” Inf. Process. Agric., vol. 4, no. 1, pp. 41–49, 2017, DOI: 10.1016/j.inpa.2016.10.005.
20) G. Pandey, R. J. Weber, and R. Kumar, “Agricultural cyber-physical system: In-situ soil moisture and salinity estimation by dielectric mixing,” IEEE Access, vol. 6, pp. 43179– 43191, 2018, DOI: 10.1109/ACCESS.2018.2862634.
21) L. He, Y. Cheng, Y. Li, F. Li, K. Fan, and Y. Li, “An Improved Method for Soil Moisture
22) Monitoring with Ensemble Learning Methods over the Tibetan Plateau,” IEEE J. Sel. Top. Appl. Earth Obs. Remote Sens., vol. 14, pp. 2833–2844, 2021, DOI: 10.1109/JSTARS.2021.3058325.
23) Y. Liu, J. Qian, and H. Yue, “Comprehensive Evaluation of Sentinel-2 Red Edge and Shortwave-Infrared Bands to Estimate Soil Moisture,” IEEE J. Sel. Top. Appl. Earth Obs. Remote Sens., vol. 14, pp. 7448–7465, 2021, DOI: 10.1109/JSTARS.2021.3098513.
24) A. Y. Kachori and K. Ghodinde, “Design of microcontroller based agribot for fertigation and plantation,” 2019 Int. Conf. Intell. Comput. Control Syst. ICCS 2019, no. Iciccs, pp. 1215–1219, 2019, doi: 10.1109/ICCS45141.2019.9065768.
25) V. Grimblatt, G. Ferré, F. Rivet, C. Jego, and N. Vergara, “Precision agriculture for small to medium size farmers - An IoT approach,” Proc. - IEEE Int. Symp. Circuits Syst., vol. 2019-May, 2019, DOI: 10.1109/ISCAS.2019.8702563.
26) M. Marie, A. Z. M. Rosli, R. Sam, Z. Janin, and M. K. Nordin, “Integrated optical sensor for NPK Nutrient of Soil detection,” 2018 IEEE 5th Int. Conf. Smart Instrumentation, Meas. Appl. ICSIMA 2018, no. November, pp. 1–4, 2019,
DOI: 10.1109/ICSIMA.2018.8688794.
27) R. Madhumathi, T. Arumuganathan, and R. Shruthi, “Soil NPK and Moisture analysis using Wireless Sensor Networks,” 2020 11th Int. Conf. Comput. Commun. Netw. Technol. ICCCNT 2020, 2020, doi: 10.1109/ICCCNT49239.2020.9225547.
28) I. Mahmud and N. A. Nafi, “An approach of cost-effective automatic irrigation and soil testing system,” ETCCE 2020 - Int. Conf. Emerg. Technol. Comput. Commun. Electron., 2020, DOI: 10.1109/ETCCE51779.2020.9350896.
Volume 05 Issue 05 MAY 2022
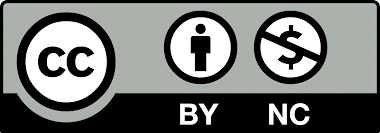
There is an Open Access article, distributed under the term of the Creative Commons Attribution – Non Commercial 4.0 International (CC BY-NC 4.0) (https://creativecommons.org/licenses/by-nc/4.0/), which permits remixing, adapting and building upon the work for non-commercial use, provided the original work is properly cited.
Our Services and Policies
Authors should prepare their manuscripts according to the instructions given in the authors' guidelines. Manuscripts which do not conform to the format and style of the Journal may be returned to the authors for revision or rejected.
The Journal reserves the right to make any further formal changes and language corrections necessary in a manuscript accepted for publication so that it conforms to the formatting requirements of the Journal.
International Journal of Multidisciplinary Research and Analysis will publish 12 monthly online issues per year,IJMRA publishes articles as soon as the final copy-edited version is approved. IJMRA publishes articles and review papers of all subjects area.
Open access is a mechanism by which research outputs are distributed online, Hybrid open access journals, contain a mixture of open access articles and closed access articles.
International Journal of Multidisciplinary Research and Analysis initiate a call for research paper for Volume 07 Issue 12 (December 2024).
PUBLICATION DATES:
1) Last Date of Submission : 26 December 2024 .
2) Article published within a week.
3) Submit Article : editor@ijmra.in or Online
Why with us
1 : IJMRA only accepts original and high quality research and technical papers.
2 : Paper will publish immediately in current issue after registration.
3 : Authors can download their full papers at any time with digital certificate.
The Editors reserve the right to reject papers without sending them out for review.
Authors should prepare their manuscripts according to the instructions given in the authors' guidelines. Manuscripts which do not conform to the format and style of the Journal may be returned to the authors for revision or rejected. The Journal reserves the right to make any further formal changes and language corrections necessary in a manuscript accepted for publication so that it conforms to the formatting requirements of the Journal.